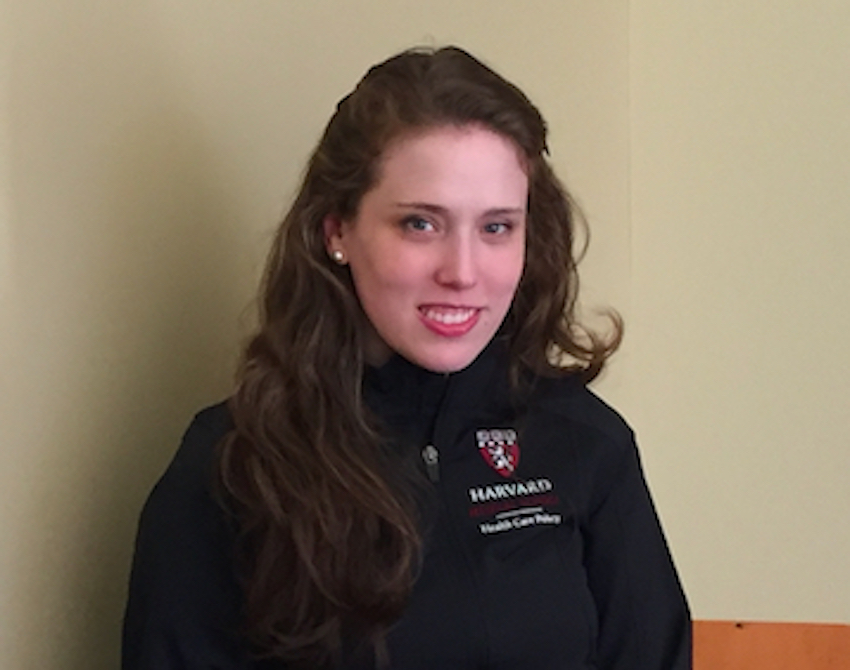
Accounting for patient characteristics is a common and critical challenge when answering questions in health policy. If a hospital treats patients with more severe medical conditions, it is important to adjust for this when assessing outcomes and quality of care at that hospital.
Likewise, when attempting to control for the fact that individuals choose their own health plans in regulated health insurance markets, researchers need to account for health status in order to draw effective conclusions about these populations.
Sherri Rose has demonstrated that new statistical machine learning methods may be better able to search the claims data used for risk adjustment in order to predict health spending. She published an article describing this work in the December issue of Health Services Research.
One finding shows that simpler formulas for plan payment discovered using machine learning are nearly as predictive as formulas that include a larger number of medical conditions. Thus, her research has implications for insurers' ability to “game” the health insurance system with aggressive upcoding of diagnostic conditions.
Rose explains these machine learning techniques in a short video, and describes computational health economics as bringing “statistical advances for big data and data science to answer critical questions in health economics."
Further work on machine learning for plan payment risk adjustment is warranted to gain a more complete picture of the impact of parsimonious formulas, including on vulnerable groups. Rose has ongoing projects with health policy PhD student Savannah Bergquist and biostatistics master’s student Akritee Shrestha tackling additional questions for both Medicare populations and those with mental health and substance use disorders.
Enjoy content like this? Sign up for our newsletter.