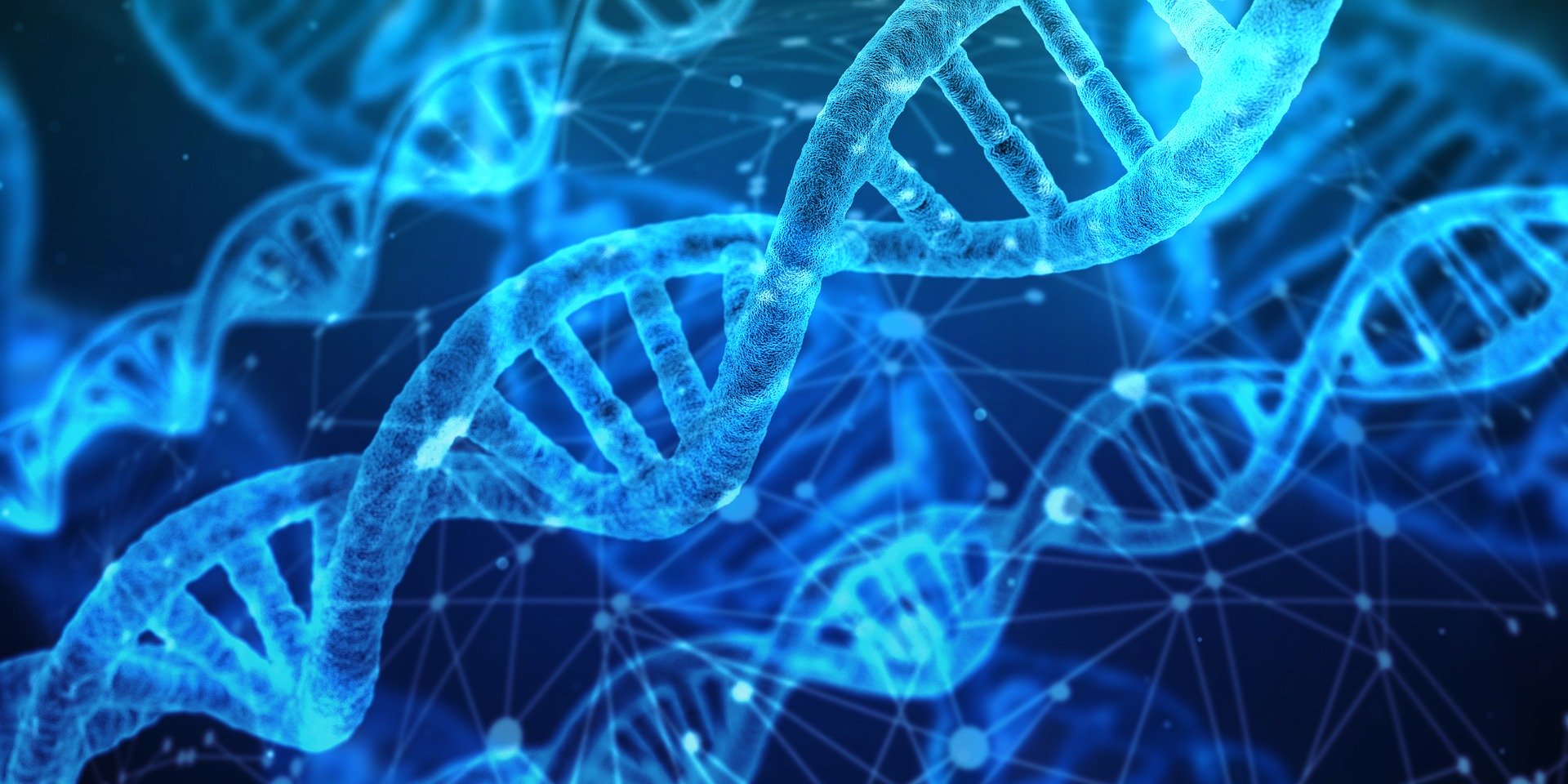
Machine learning tools are important yet underutilized techniques in health services research. In the International Journal of Epidemiology, associate professor of health care policy (biostatistics) Sherri Rose, PhD, discusses how machine learning can benefit health services research, and how it intersects with epidemiological methodologies.
While machine learning is not currently widely used in health services research, it is becoming more prominent in areas such as health care spending, outcomes, and quality. Epidemiological expertise can bolster the effectiveness of this research when applied collaboratively. Many recent studies focus on predicting outcomes, with fewer examining questions in causal inference, such as the impact of a policy or the comparative effectiveness of multiple treatments. Causal queries in particular can benefit from leveraging epidemiological methodology when incorporating machine learning.
“Health services research as a field is less flashy than many domains publicizing dramatic advances using ‘artificial intelligence’ methods,” Rose says, “but this is not to say that careful, reasoned machine-learning work will not lead to progress in improving health care costs, quality, access, [and] outcomes.”
As machine learning in health services grows, it will most likely include methodology for difference-
in-differences study designs. These studies will continue to benefit from added epidemiological expertise.
Rose was interviewed about this research recently on the TWIML AI podcast.